Machine learning has moved beyond the realm of academic research and into practical, real-world application across a wide range of industries. Whether it’s automating customer service, predicting inventory needs, or optimizing marketing campaigns, machine learning can unlock valuable insights and increase efficiency. But purchasing and implementing machine learning algorithms for your business is not as simple as buying a piece of software—it requires strategy, planning, and a clear understanding of your business goals.
In this guide, we’ll walk you through the steps to effectively purchase and implement machine learning solutions that align with your company’s needs.
1. Understand Your Business Needs
Before jumping into vendor comparisons or tech jargon, take a step back and assess what you’re trying to accomplish. Are you looking to improve customer engagement? Automate repetitive tasks? Reduce churn or increase conversion rates?
Clearly defining your objectives will help you choose the right type of machine learning solution, whether it’s a pre-built model, a platform for creating custom solutions, or a consultant-based approach. Some common use cases include:
- Predictive analytics – Forecast customer behavior or demand.
- Natural language processing – Analyze customer feedback or support tickets.
- Recommendation engines – Suggest products to users based on past behaviors.
2. Choose Between Off-the-Shelf vs Custom Solutions
When shopping for a machine learning algorithm, you’ll generally face two options:
- Off-the-shelf solutions: These are pre-built tools or services that can be quickly integrated into your business operations. They are best suited for common problems, such as image recognition or fraud detection.
- Custom solutions: Developed specifically for your business, these generally provide better performance for unique challenges but take longer and are more expensive to implement.
Startups and small businesses often find off-the-shelf tools valuable due to their low cost and quick deployment, while larger enterprises may benefit from the flexibility of custom-built models.
3. Evaluate Vendors Carefully
Once you’ve narrowed down the type of solution you need, start researching potential vendors. Compare features, scalability, pricing models, and user support. Ask about:
- Data requirements and security features
- Integration capabilities with your existing systems
- Training and support options
- Proven case studies or testimonials
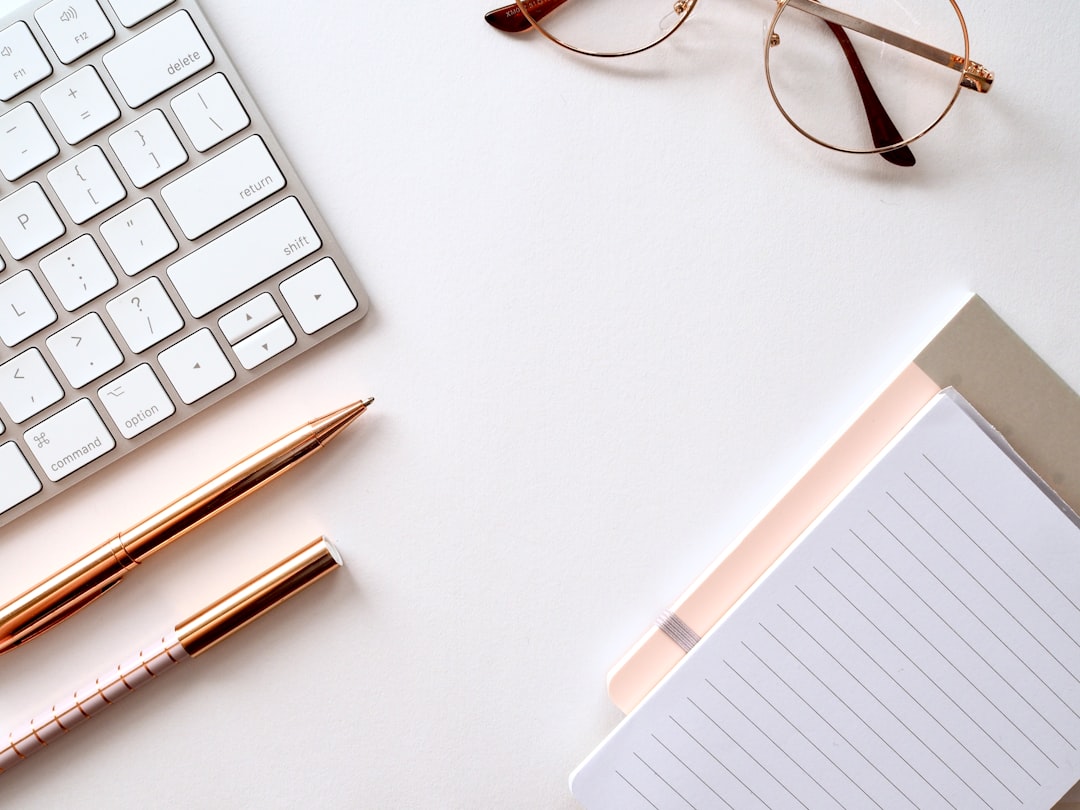
Don’t just focus on the technical specs; consider the vendor’s expertise in your industry. A vendor with domain-specific experience can offer insights and best practices that can fast-track your success.
4. Begin With a Pilot Project
Before rolling out a machine learning solution across your entire organization, conduct a small-scale pilot. Choose a single use case with clearly defined success metrics. This allows you to:
- Validate model accuracy and performance
- Identify integration challenges
- Gather user feedback and adjust accordingly
A pilot helps mitigate risks and ensures your team becomes familiar with the system before larger deployments. From the pilot, you can refine your approach, improve data quality, and streamline workflows.
5. Invest in Data Quality and Infrastructure
Machine learning is only as good as the data it relies on. Clean, structured, and comprehensive datasets are critical to generate meaningful results. Ensure that your data infrastructure can handle the demands of a machine learning system. This includes:
- Data warehouses or lakes for storage
- APIs and connectors for real-time data flow
- Proper labeling and annotation tools
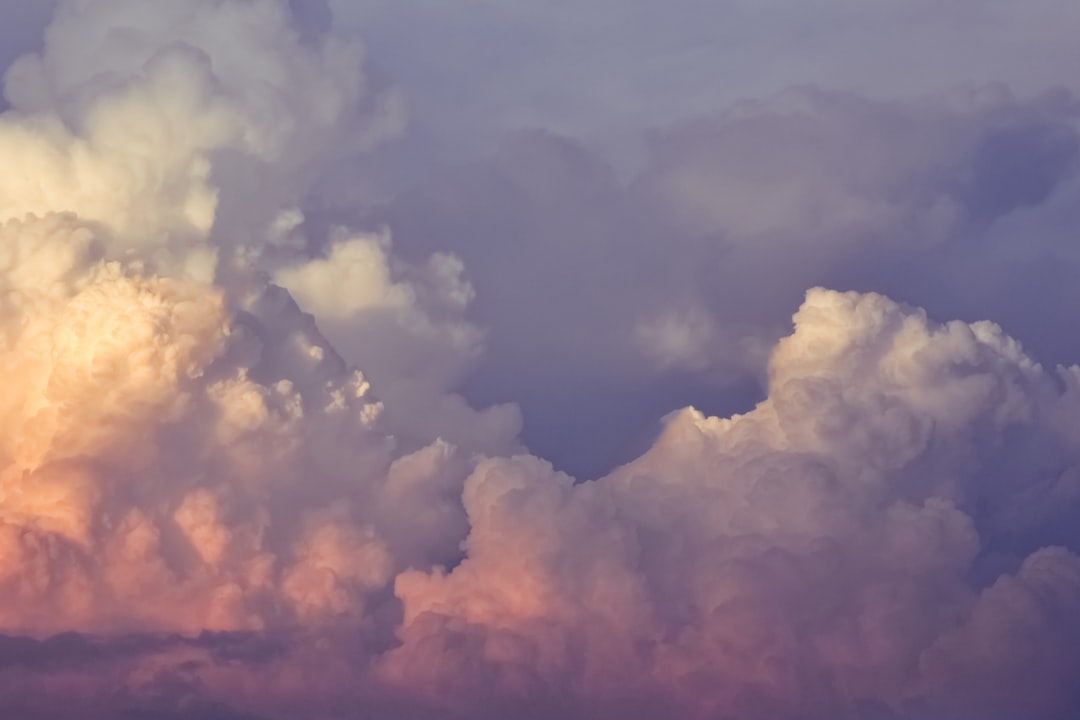
Collaborate closely with your IT team to ensure systems are robust, scalable, and secure.
6. Train Your Team
Introducing a machine learning system into your business isn’t just a technical change—it’s a cultural one. Your staff will need training on how to interpret model outputs, integrate them into decision making, and provide feedback to improve the models over time.
Consider offering workshops, partnering with consultants, or leveraging online platforms to build internal capabilities. Building in-house knowledge ensures long-term sustainability of your ML initiatives.
7. Monitor and Optimize Continuously
Machine learning models are not static—they must be monitored, evaluated, and retrained as new data and business needs evolve. Set up a schedule to track key performance indicators (KPIs) and conduct periodic audits.
Questions to consider include:
- Is the model performance degrading over time?
- Are there signs of bias or inaccuracies?
- Can newer datasets improve the model?
Continuously refining your algorithms ensures they remain effective and aligned with your objectives.
Conclusion
Purchasing and implementing machine learning algorithms for your business isn’t just about acquiring software—it’s about reshaping workflows, improving decision-making, and enabling innovation. By understanding your needs, evaluating the right solutions, and investing in data and people, you’ll be well-positioned to harness the power of machine learning competitively and responsibly.